AI-enhanced design unveiled for industrial processing equipment in Birmingham
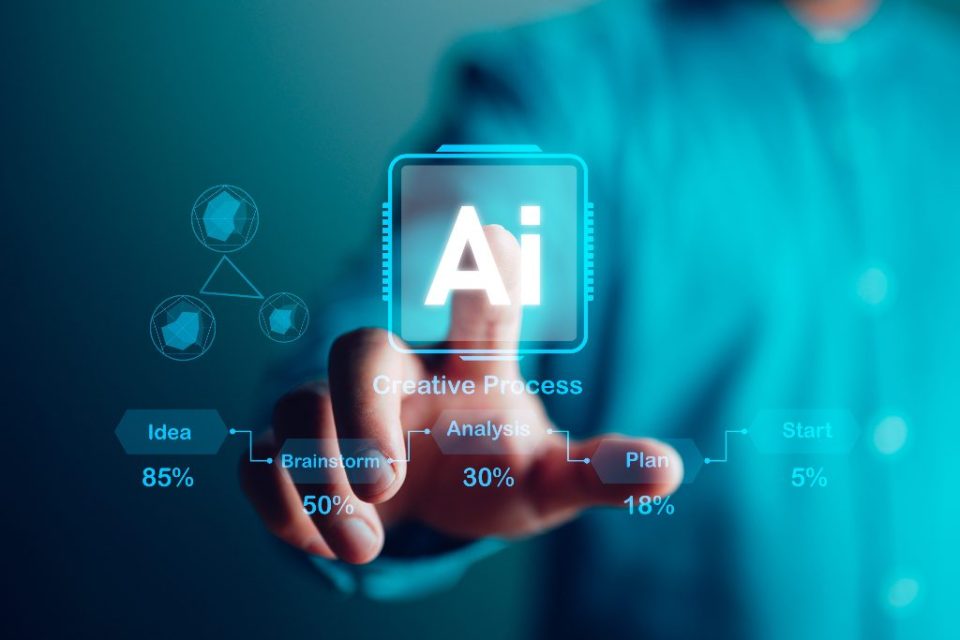
EvoPhase is set to employ an AI-driven ‘evolutionary design’ approach that is relevant to a wide variety of equipment spanning multiple industries.
The University of Birmingham Enterprise is pleased to introduce EvoPhase, a service-oriented venture dedicated to optimizing both existing and newly developed process equipment used for mixing, blending, storing, or agitating granular materials.
EvoPhase will utilize evolutionary AI algorithms alongside simulations of particulate behavior within systems such as industrial mixers. These technologies will be used to iteratively refine the design of mixing blades, as well as the shape and size of blending vessels.
This AI-guided ‘evolutionary design’ methodology can be applied to a diverse array of process equipment, encompassing mills, dryers, roasters, coaters, fluidized beds, stirred tanks, and is anticipated to yield substantial cost and energy savings for various industries.
Our technologies enable us to undertake assignments in material characterisation, digital model development, experimental imaging and validation, optimisation of process conditions, geometric design optimisation and scale-up, and predictive model development. Our approach is suitable for designing powder, granule and fluid processing equipment across all industries, where it will deliver cost savings by increasing energy efficiency, mixing effectiveness and throughput said Leanard Nicuson, Chief Technology Officer, EvoPhase.
The Chief Executive Officer, Dominik Werner, the Chief Technology Officer, Leonard Nicusan, the Chief Operating Officer, Jack Sykes, and the Chief Scientific Officer, Dr. Kit Windows-Yule, hail from Birmingham’s School of Chemical Engineering. All four possess extensive experience in digital modeling and simulations of industrial processes. Their collective expertise will empower EvoPhase to tackle challenges that conventional R&D approaches often find difficult to overcome.
Up to 50% of the world’s products are created by processes that use granular materials, but granules are difficult to characterise or understand. If you consider coffee, its granules are solid when they are contained, like a liquid-like when poured out of the container, and become gas-like and dispersed if you blow on them. This type of variability means granules are the most complex form of matter to process said Dominik Werner, CEO, EvoPhase.
The team is set to harness a groundbreaking AI technology named Highly-Autonomous Rapid Prototyping for Particulate Processes (HARPPP), which operates in a manner reminiscent of the natural selection process. HARPPP systematically evaluates evolved designs to pinpoint the optimal solution. Notably, it empowers users to configure multiple parameters for optimization, fostering the evolution of a design that can simultaneously meet diverse objectives, such as minimizing power consumption, maximizing throughput, and optimizing mixing rates, without necessitating trade-offs between these parameters.
Furthermore, EvoPhase will integrate a numerical methodology known as the Discrete Element Method (DEM). DEM predicts the intricate behaviors of granular materials by meticulously modeling the movements of individual particles. These intricate simulations can be substantiated and refined through the use of Positron Emission Particle Tracking (PEPT), a pioneering technique originating from Birmingham. PEPT represents a variant of the medical imaging methodology known as positron emission tomography (PET).
Leonard Nicusan said: “Our technologies enable us to undertake assignments in material characterisation, digital model development, experimental imaging and validation, optimisation of process conditions, geometric design optimisation and scale-up, and predictive model development. Our approach is suitable for designing powder, granule and fluid processing equipment across all industries, where it will deliver cost savings by increasing energy efficiency, mixing effectiveness and throughput.”